Tony Alves, SVP of Product Management, attended the STM US Annual Conference in Washington, DC, April 24 and 25, 2024. The meeting centered on the transformative impact of artificial intelligence and digital technologies on the landscape of scholarly publishing and communication. Key themes included the integration of AI as a core component of scholarly practices, enhancing collaboration between humans and machines, and addressing the infrastructural needs of the digital transformation in publishing. Discussions explored the ethical, transparency, and trust challenges posed by AI, emphasizing the need for maintaining integrity within the scholarly community amidst technological advancements. The conference also highlighted the evolving roles within research offices, libraries, and editorial practices, reflecting on how AI could optimize workflows, enhance data management, and potentially reshape future research dissemination. The sessions collectively underscored a proactive approach towards embracing technological innovations while safeguarding the core values and reliability of scholarly outputs.
Tony’s report is in three parts. In this final installment he reports on a very forward-thinking journal editor who is embracing technology. Tony also summarizes a presentation that discusses how publishers might approach changes in technology and embrace some different ways of thinking.
The Journal Editor: A view from the future
Darren Roblyer, Associate Professor, Boston University
Focus: Investigating the impact of generative AI on the roles and processes within the journal editing and peer review landscape.
Key Points:
- Current use and future potential of AI in automating tasks traditionally performed by human editors, including manuscript drafting and peer review.
- Challenges related to AI integration, such as maintaining the quality and integrity of scholarly outputs.
In the future landscape of publishing, generative AI is becoming increasingly commonplace, reshaping the roles and processes within the industry. Currently, 30% of publishers use AI to write papers, and 15% utilize it for peer review purposes. A significant challenge arises in detecting AI’s involvement, as it becomes more sophisticated, making its detection increasingly difficult.
A study analyzing 146,000 peer reviews from prominent AI conferences—International Conference of Learning Representations, Conference on Neural Information Processing Systems, and Conference on Robot Learning—revealed that up to 17% of peer-review reports had been substantially modified by chatbots like ChatGPT, especially when reviewers were pressed for time. The study noted that AI tends to introduce a positivity bias and utilizes certain adjectives more frequently than humans.
In response, major scientific journals such as Nature and Science have established policies that prohibit the use of generative AI in peer review, requiring any AI involvement to be disclosed. Despite these guidelines, the risks associated with AI in publishing are notable. AI-generated texts often lack specific feedback and fail to properly cite other works, sometimes even “hallucinating” citations. Moreover, these texts tend to reduce linguistic variation and epistemic diversity, potentially amplifying existing biases and raising accountability issues.
However, the integration of AI also presents several opportunities. AI can improve initial submission screening processes by assessing fit to the journal’s scope, checking the length and grammar of submissions, and verifying statistical presence. It enhances language and grammar quality, checks citation accuracy, and assists in peer review processes, potentially improving the overall quality of papers and reviews. Publishers are also starting to leverage their past reviews to enhance the functionality of AI systems, moving towards more interactive and dynamic forms of academic papers.
Looking ahead to 2034, one can predict significant changes for a typical journal. All papers will have associated data, many with accompanying code, and robust databasing will be a standard practice through partnerships with repositories. An increase in preprints is also expected, reflecting a more open and accessible research process.
In terms of analytics, traditional metrics like citations and downloads will be supplemented with detailed insights into readership patterns. AI will play a central role in manuscript writing and reviews, with stringent protocols for disclosure and confidentiality. It will also be employed to pre-check manuscripts, assist editorial decisions with AI-generated peer review reports, and verify citations and plagiarism.
Manuscript types will evolve, with more meta-analyses and experiments with interactive articles that incorporate AI directly into the publisher’s platforms. Content will become more adaptive, featuring new visualizations and real-time updates. Enhanced accessibility will make research more inclusive, increasing the journal’s reputation and community engagement.
Institutional incentives for tenure and promotion will shift focus from traditional impact factors to broader impact metrics. AI integration will emphasize the value of data generation over writing skills, and some predict a move away from the traditional peer review and PDF models towards a more fluid and continuously editable format of scholarly communication. This future envisions a publishing landscape that is deeply integrated with AI, continuously evolving to better meet the needs of the scholarly community and broader society.
How do we move to the future?
Todd Carpenter, Executive Director, NISO
Anita de Waard, VP Research Collaborations, Elsevier
Focus: Debating the strategic application of AI in scholarly communication, weighing the benefits and challenges of point solutions versus platform solutions.
Key Points:
- Discussion on the immediate benefits of point solutions and the broad, systemic changes enabled by platform solutions.
- Considerations for adopting AI technologies in a manner that supports sustainable and ethical scholarly communication.
The book “Power and Prediction, The Disruptive Economics of Artificial Intelligence” by Ajay Agrawal, Joshua Gans, and Avi Goldfarb, explores the concepts of point solutions versus platform solutions within the context of artificial intelligence.
Point Solution is described as a targeted approach that solves a specific problem without considering related issues. This type of solution is popular for its ability to address an immediate need or implement a new service rapidly. Point solutions are particularly useful for quick fixes or specific enhancements within an existing workflow, providing immediate benefits without the necessity for major systemic changes.
Platform Solution, on the other hand, refers to a foundational technology that supports broader changes to entire processes or workflows. Unlike point solutions, platform solutions are designed to be scalable and adaptable, allowing for ongoing improvements and innovations that can impact multiple aspects of an organization or system. This type of solution is strategic, aiming to provide a base upon which various functionalities can be built and integrated, facilitating a more holistic approach to problem-solving and development.
While point solutions focus on addressing immediate and specific problems, platform solutions provide a comprehensive foundation that enables extensive and continuous improvements across various facets of a workflow or system.
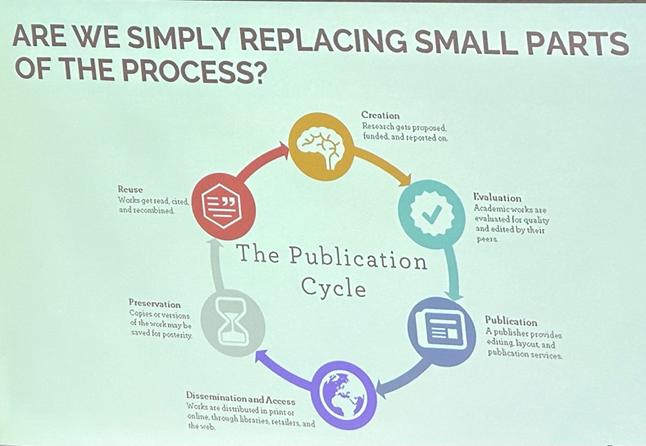
The traditional method of writing and reading research articles involves a linear and structured process. Typically, articles are crafted starting with a clearly defined Problem or Goal based on previous research findings. Researchers then select appropriate Methods to test this hypothesis and document the Results. These results yield Data, which forms the basis for making an Assertion about the hypothesis, often supported by statistical evidence.
When reading these articles, the process is equally methodical. Readers begin by examining the Assertions made in the article, then analyze the Results that support these assertions. The Methods section is scrutinized to verify the appropriateness and reliability of the techniques employed. Finally, readers consult the References to check the validity of the methods and results and to contextualize the findings within the broader scientific discourse.
In contrast, one proposed alternative methods for reading research articles introduce more interactive and interconnected approaches. The presenter suggests a Knowledge Network model where research findings are visualized as part of a broader network, such as a cellular pathway model. Here, Assertions from the literature are directly linked to the underlying Evidence from various experiments. This networked approach allows readers to see how different pieces of evidence connect within the larger framework of existing knowledge, enhancing the understanding of complex scientific relationships.
Another approach, suggested by the presenter, centers around a Database of Experimental Outcomes. This database would contain normalized data from multiple research articles, with Individual Results linked to their specific experimental outcomes. These are then contextualized with relevant information including the data, problem tackled, methods used, and assertions made. Such a structured database allows for systematic access to research data, facilitating easier comparison and contrast across different studies, and simplifying the process of integrating new findings into existing knowledge bases.
These alternative reading methods differ significantly from the traditional linear approach. They offer a more dynamic, interactive way of engaging with scientific literature, potentially increasing the efficiency and depth of research reviews. This can improve the ability to evaluate the reliability of results and integrate new findings more effectively into the broader scientific context.
– By Tony Alves